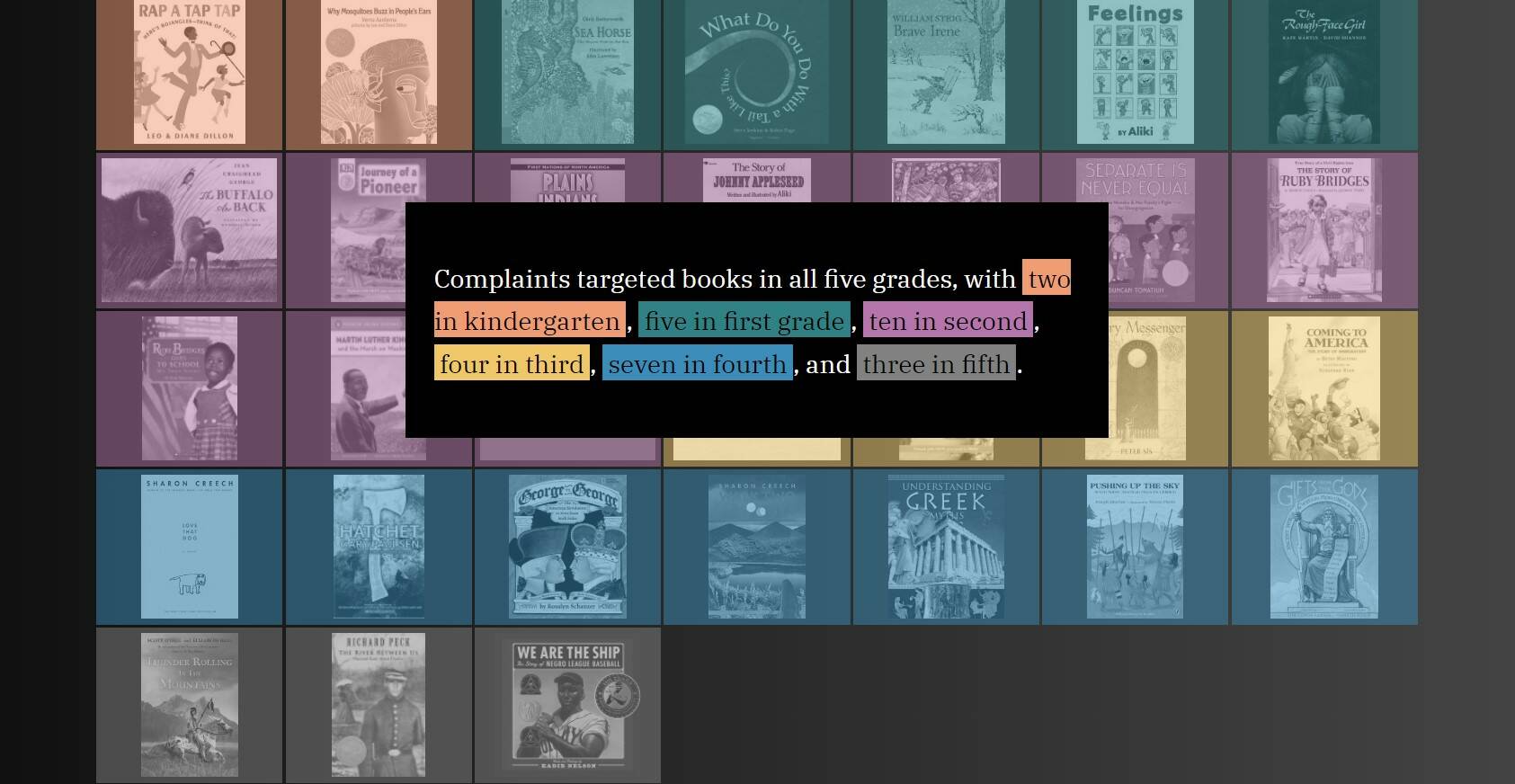
How we turned an elementary school curriculum challenge into a dataset
An editorial approach to creating data visuals from text-based reports
Last year, Tennessee enacted a law intended to restrict K-12 classroom discussions about the legacy of slavery, racism, and white privilege. Then Williamson County Schools, a district south of Nashville, received forty complaints about texts in its K-5 English language arts curriculum.
The district later released a 113-page report that detailed the challenges, their outcomes, and the decision committee’s reasons.
Chalkbeat reporter Marta W. Aldrich found two Williamson parents, numerous experts, and teachers to dig into the county’s curriculum debate, which often circled the idea that some texts were simply “age-inappropriate” to teach in early elementary school. These voices captured the human aspect of the story, as well as the conversations around policy and educational practice.
But we also wanted to analyze the complaints themselves. It was a matter of fact-checking, as well as adding crucial context. A giant report like this is rich with data, if you can decide how to convert it from plain text into a structured dataset.
We wanted to quantify: Who has challenged the books? Why? What were common themes and phrases? Did the complaints’ contents actually line up with what experts and parents are observing in the story?
The answers became the introductory visualization in our final story, How the age-appropriate debate is altering curriculum in Tennessee and nationwide.
The viz juxtaposed concrete data against the euphemistic talking points that have become common in covering curriculum debates. This analysis equipped readers with the knowledge of what, exactly, sources were talking about (or around) in the following story.
Working backwards from tables and semi-structured data
The report offers a few obvious starting points: data viz! Tabular data lets us start building a dataset of books challenged, the grades they’re taught in, and the challenge outcome.
The report also contains a few other tables, including breakout aggregates of the outcome results by status and a list of books that were adjusted or removed.
Even better, each individual book has a full page evaluation report, which we can use to add more detail to the committee’s decision-making process. Particularly interesting: categories indicating whether books were on topic, valuable for educational purposes, or objectionable. This also allowed us to fact-check the tabular data up above. (We caught two discrepancies and were able to reconcile them by comparing the tables to the individual report pages.)
All of these are great! They are the starting blocks for a structured dataset that contains some basic information on the challenges and their outcomes. But, overall, it doesn’t tell us much about what’s actually in the books, why parents filed complaints, or how the committee evaluated the texts.
Finding themes
Instead, we started looking at the text itself. There are programmatic ways to do this using keyword extraction, but it was simple enough to manually catalog thirty books laid out in one consistent document format.
We debated digging into the more detailed complaints, which are appended to the main summary documents. The detailed complaints had a few advantages: they were original written complaints, rather than summaries written or quoted directly through the appeal board, and they contained more expansive comments about specific pages or lines.
However, when we attempted to analyze those complaints, they were (unsurprisingly, for a public comment process) often unclear or vague about their objections. Many addressed teachers’ materials that aren’t published with the books; we could not consistently access those. Some complaints just seemed random or irrelevant. For instance, one complaint noted, “It’s all about changing seasons & Chamaeleon,” to which the committee responded, “The committee does not share the concern of the complainants.” Another simply read “CA,” to which the committee responded, “The committee does not understand this concern.”
In other words, lots of junk data.
So instead, we focused on the committee’s summaries. Though they carried the downside of an added filter, the committees did directly quote the complaints, and they had already done the work of identifying items relevant to the curriculum.
I added booleans for keywords as we encountered them — “agenda,” “conditioning,” “oppression” — and summarized other themes or concepts that materialized — “negative about white people,” “age inappropriate,” “dark or scary.” A few themes showed up once (“boring to boys”) but never came up again, whereas others reappeared consistently.
We also tracked themes in the outcomes sections. I created several text and note sections, then boiled them down into a few more booleans based on the committee’s defense of each book (“historical value” or “taught in context”) and the ultimate outcomes (“language changes during teaching,” “omit pages,” “make counselors available.”)
With a full dataset of trends in front of us, it was easier to see patterns in the complaints.
This also gave us an easy plain-text way to send the findings to reporters and editors. They were able to review what we left out, then recommend including or excluding other items based on how the story’s reporting developed.
As I decided what to highlight, I asked a few questions:
What’s the basic background that we need to add?
This became the books broken out by grade level, an overview of the residents who filed the complaints, and the ultimate outcomes.
What information is necessary to understand the broadest themes of the data?
This became a few of the filters, including some elements that weren’t foregrounded in the main story. For instance, sexual themes and violence didn’t really come up in the reporting, but the complaints mentioned them regularly, and they often coincided with complaints about “age inappropriate” discussions of race — so it seemed important to include.
These themes, taken as a group, also starkly emphasized the difference between common explanations for book bans and the actual content of the challenges against the books. For instance, books challenged specifically for containing “critical race theory,” being age-inappropriate, or being racist included two historical accounts of Ruby Bridges attending an all-white elementary school as a black child in New Orleans at age 6. (One of those books was her autobiography.)
The Williamson County committee summarized what they heard from parents in the hearing: “Themes of segregation and racism are not appropriate for this age group or grade level in which 7–8-year-olds do not yet have the maturity or capacity to think critically.” A book about Sylvia Mendez’s elementary school experiences received similar commentary.
Though we couldn’t capture all that detail in the viz, it was important to describe the core: “Parents accused three of those books of being racist because they depicted racism. Those books each recounted historical fights to desegregate schools.”
What details are interesting or notable within the context of the story?
We ended up excluding a lot of the details on how the committee justified their decisions, because they just weren’t that remarkable on their own. But the recommendation to make counselors available struck us as worth including, especially because the books that received that recommendation were somewhat surprising.
We also highlighted some rarer complaints. For instance, books depicting the U.S.’s historic mistreatment of Native Americans and immigrants were accused of being “anti-American,” which only came up in relation to three books. But these phrases seemed particularly relevant to broader conversations about race and how it is taught, so we included them.
We also ended up ignoring flags that might have seemed initially like they should be included. For instance, the phrase “critical race theory” seems like it would be relevant to a story on curriculum restrictions for “critical race theory.” However, the specific phrase only came up four times. And in two instances, it was used in confusing (and arguably definitionally incorrect) ways. Most complaints used vaguer language about “hate” and “division” to refer to similar ideas.
Wrapping it up
At its core, this is just due-diligence reporting. Plus, treating the review outcomes as a dataset gives us a systematic way to look at a curriculum challenge. Though there are ways to proceduralize this kind of keyword search-work, it didn’t take long to read the entire report.
But it’s also important to take these extra steps to dig into the actual content of the texts. A lot of coverage over curriculum debates has leaned heavily on soundbites, without exploring the exact educational materials being challenged under euphemisms like “age-inappropriate” or “divisive.” By digging into those complaints as data, we hope to model a more accurate methodology for culture-war reporting.