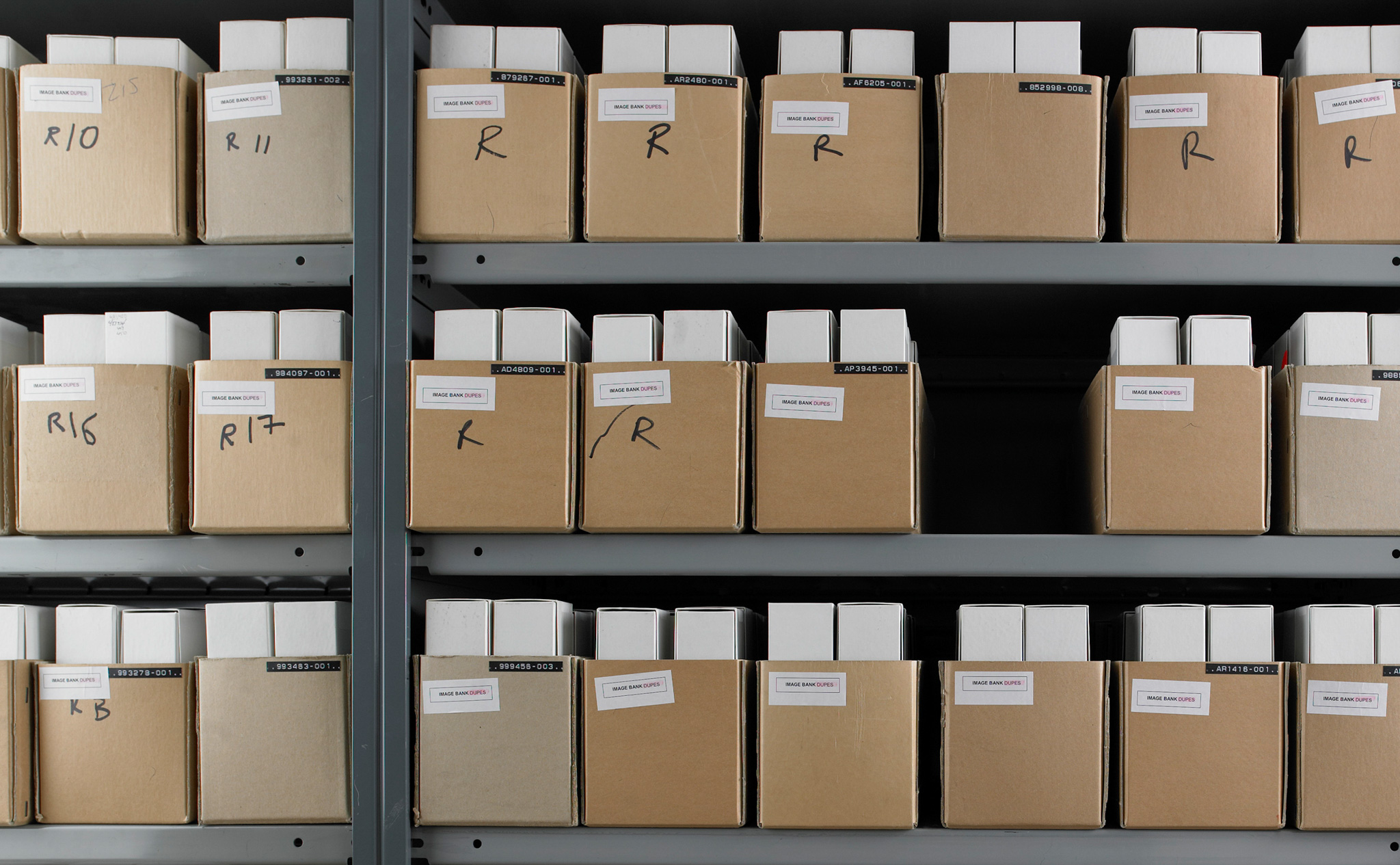
Showing what's not there
How to responsibly visualize flawed data — and when to not
Municipal and state data is often imperfect. At its best, it’s riddled with suppression, missing values, or missing important categories or identifiers. At its worst, agencies export and maintain it incorrectly — it’s full of duplication errors, or an Excel formula got dropped or deleted, or there are 1,600 columns with unclear names and no documentation. That’s assuming you can even obtain it in a usable format, instead of trying to drudge meaning out of a PDF of slapped-together JPEGS.
These problems are only compounded the moment that you need to compare anything between cities or states, each with their own distinct problems.
Sometimes, analysis and text are sufficient and give you room for necessary disclaimers about data quality. Others, even extensive cleaning, clever restructuring, or other more intensive troubleshooting can’t change that the data has some fundamental, underlying issues.
If you’re trying to then visualize this data, you run into more problems. A chart, even with a lengthy note, communicates certainty in a way that a caveat-filled paragraph doesn’t. Audiences tend to take an image at face value.
Here are a few ways that Chalkbeat navigates this.
Showing non-comparable data on the same topic
Okay, so you’ve got a bunch of data. It’s pretty interesting data! No one else has put it all together! Assembling it would be novel work that could shed light on an important issue! But there’s no standardized tracking, not every agency even has it, and the agencies that do have it define it all differently.
You can’t let audiences compare it. It’s not comparable.
Which means you can’t put it all on the same axis.
What next?
Don’t let people compare the data
One solution: small multiples. This is the great way to show the landscape and variability in data without encouraging people to draw conclusions that just don’t exist.
If you want to, you can also add visual flags, notes, and other cues to emphasize just how different the data is.
Here’s one way Chalkbeat used this method to collate teacher retention data, which has been a subject of much debate in the education world:
You’ll notice that instead of laying out the line charts side-by-side, which we do more often when the data is at least slightly consistent but topically different, we require an interactive step to switch between datasets.
This graphic has a few key advantages:
- The one-view-at-a-time format prevents audiences from comparing data that doesn’t use the same time-frames, collections processes, and definitions.
- That extra “interaction” step cues audiences that they’re switching into something that is different, even if they don’t understand the full extent of how or why.
- Individual notes let us define and describe the data on a case-by-case basis.
Show how and why it can’t be compared
But if the data is so not-comparable, or has additional collection and methodological issues, you might not even be able to do that.
In that case, it might be worth a graphic that highlights the uneven landscape — and the sheer impossibility of reporting all the data. You could highlight the different criteria for collection, states that don’t release data, school districts where a given survey wasn’t distributed, and so on.
We did that here:
This graphic doesn’t even dig into the fact that there are half a dozen different definitions of “nonbinary student” in this data, so those states that do collect it may be categorizing different groups of students.
But, it highlights the uneven landscape: a mix of released data, unreleased data, and non-collection at the state level. It also neatly sidesteps the issue that the actual data itself is pretty mediocre.
A similar concept is also applicable anywhere that measured reporting thresholds are different, or where municipal data doesn’t make it up to state or federal reporting due to noncompliant collection methods (or just plain shoddy reporting).
Showing suppression and other missing data
When there are systematic flaws in something we want to chart, the solution is often to go with a really limited visual that doesn’t require using the problem data. This often involves abandoning over-time trends and other common visual approaches.
However, if you get a bit creative, you can analyze and visualize the quality of the data instead of the content of the data. This is especially appropriate when the poor-quality data illuminates an accountability failure.
Show what we do have
The simplest solution, of course, is to work around the data.
When we wanted to visualize the tiny handful of students reported as nonbinary by state departments of education across the U.S., we ran into a few issues. Different timeframes, for one. But also, because the student populations were so small, the over-time trend data was tiny at the absolute level, and extremely subject to looking like it had huge surges at the proportion level (from, say, 12 to 38 kids — a 217% increase that accounts for less than 0.1% of a state’s K-12 population).
As an extra fun problem, some school districts across the same states started letting students change their gender markers to nonbinary at different times — and others might not even have a way for students to report that data, even though the state has started collecting it. So the actual reporting entities change quickly year-over-year. On the flip side, other states added the option all at once at the state level, but students might not know it existed for a year or so.
This poses extra ethical issues due to widespread misinformation about increases in populations of trans youth. A misstep in visualization could enable bad-faith, out-of-context social media screenshots and contribute to viral misinformation.
So, we removed the trend data in favor of first-year and most-recent-year snapshots.
This restriction controls for the major problems with the data, but still gives some idea of the current reporting landscape.
On the other hand, when dealing with student testing data for online schools in Colorado, it simply didn’t make sense to do that — the snapshot data and the trend data were both bad because many of the schools were so new, and eligible students weren’t taking the tests. That meant that even though a lot of the data was reported, it wasn’t really meaningful or representative.
Instead we just chose a metric that every school did have to report for all students: graduation rates.
This isn’t always an option, of course, but especially when you’re working with big state releases, there may be multiple datasets that answer the same question.
In our case, we just wanted to know, “how do the students at online schools perform compared to students at brick-and-mortar schools?” Any number of datasets could answer that question. We just went with the one that showed the most complete picture of student outcomes, instead of the more traditional test score metric.
Show what we don’t have — and how prevalent that is
If all else fails, sometimes it’s better to visualize the information that is missing — demonstrating concretely what’s there and what’s not.
On the Colorado online schools story above, we found that we weren’t the only people having problems evaluating the performance of the schools. The schools threw up a bunch of “could not evaluate due to incomplete data” flags for state education department analysts, too.
The state releases categorized performance information each year. This ended up serving as a nice shorthand, which we were able to use to show the full extent of how little insight anyone has into the performance of these schools. It also emphasized that this problem was unique to these schools.
But in other instances, no one is doing that calculation for you. Still — you can do it yourself.
In the case of this government dashboard in Indiana, that’s what we ended up doing. The Indiana Department of Education started tracking median income of high school graduates in one of several attempts to understand student outcomes. In the dashboard, the data looks straightforward. And it’s presented as a comprehensive income tracking resource.
But if you check the data definitions, there’s a red flag: the median is based only on students who have “sustained employment,” which itself has three definitional requirements.
Okay, so maybe we can show general employment and sustained employment?
Except… how is this data collected? Well, it’s based on workers… employed only in Indiana… and whose employers participate in unemployment insurance. Which means that any graduates who move out-of-state aren’t tracked at all. And not even all workers in Indiana are tracked, on top of the “sustained” vs. more general employment definitions.
So instead, we FOIA’d the raw numbers, did some simple subtraction, then converted to portions show exactly how incomplete the data is:
It really emphasizes how unrepresentative the median is — and doesn’t even require explaining all the complicated limitations of the dashboard.
Knowing when something’s just too bad to work with
It’s of course also important to consider when data is so bad that you might take on liability by trying to use, fix, or interpret it. And it’s extra important to consider when visualization might lend credibility to trends that speak more to the randomness of bad data than any sort of reality.
Large margins of error caused by incomplete or suppressed data are an obvious one. In education data, for instance, I frequently check the suppressed totals against the unsuppressed totals, and make sure that the portion of unreported student data in any subselection of schools doesn’t exceed a reasonable threshold. If a third of relevant students’ data is omitted, it’s probably not a very useful metric.
But there are subtler signs, too.
If a data collection is new, year-over-year trend data might be complete but subject to the whimsies of inconsistent implementations, slow rollouts, and spotty instruction on how to collect and report the data, especially if the data deals with a small subpopulation. If the data definitions changed for only one year — as we see frequently with COVID-19 data — it may be reasonable to make that one year visually distinct, but if the data definitions change every year, or multiple years in a row, a visual trend is just noise.
Maybe you’ve assembled data from multiple states or regional agencies that all track roughly the same thing but use totally different definitions, or are staffed inconsistently for in-person data collection like inspections. In that case, the data might be usable on an individual basis, but any assembled dataset could imply comparisons that just aren’t there.
Deciding what’s too bad is often more of an editorial call than an exact science, but the core questions to ask yourself include:
- Is so much data missing that any visualization distorts reality?
- Is it possible that the visual will overstate random noise, or accidentally create the impression of a trend that may or may not exist?
- If new agencies, geographies, domains, etc, are being introduced to the tracking, is there any way to normalize for it — e.g. with a per capita calculation or some other metric?
- How much would we need to explain this to an audience? Is it possible to explain it clearly and accurately, or would any data work be so full of caveats that it renders the important information incomprehensible?
- As a journalist, are you comfortable standing by and defending the judgment calls you made to interpret the data?
Why and when is this worth all the time and effort?
It’s also worth considering whether the data’s low quality makes the story extra important.
Sometimes in newsrooms, if you pitch a data story with a million caveats attached, an editor’s response is: We can’t run a story if the data’s no good.
It’s a reasonable first impulse, in some senses. But it’s also a major oversight from a journalistic perspective: What’s the point of a free press, if we’re not uncovering new information and shedding light on problems that the public has overlooked?
In an increasingly data-driven age, problems with data are problems for people.
For instance, though the U.S. Census and other data reporting on multiracial people is notoriously inconsistent, they’re a growing portion of the U.S. that shouldn’t be ignored. And oversights in data collection can have significant impacts for individuals, because it can impact research and funding. Entirely missing data also means that government oversight measures can’t be enforced.
Part of the reason we encounter this so often at Chalkbeat is that, in part, we’re a mission-driven publication that covers public education from an equity-focused lens. That means we’re specifically interested in showing what’s happening to students who have been historically underserved by their public schools. And that often means that our data work tries to look at students, schools, and issues that are omitted or overlooked not just by other journalists, but by the systems that track and create data on schools to start with.
So when there is data that’s flawed, but it’s on especially undercovered groups, or on an issue that is complicated to track, it can be well worth the time to figure out some way to report within the limitations of the existing data — as long as you keep in mind why it matters, and who it matters to.